Build Real-Time Knowledge Graphs for AI Agents
RAG can’t keep up with real-time data.
Graphiti builds live, bi-temporal knowledge graphs so your AI agents always reason on the freshest facts. Supports semantic, keyword, and graph-based search.
100% open-source with 5,000 stars.
GitHub repo (don’t forget to star the repo) →
Thanks to the Graphiti team for partnering today!
Knowledge Distillation using Teacher Assistant
Knowledge distillation is quite commonly used to compress large ML models after training.
The idea is to train a smaller/simpler model (called the “student” model) that mimics the behavior of a larger/complex model (called the “teacher” model):
Today, let’s discuss a “Teacher Assistant” method used to improve this technique.
Let’s begin!
If you want to get into more detail about the model compression, we covered 6 techniques for model compression here: Model Compression: A Critical Step Towards Efficient Machine Learning.
An issue with knowledge distillation
Ideally, the student model should retain the maximum knowledge of the teacher model while being as small as possible.
However, in practice, two things are observed:
For a given size of the student model, there is a limit on the size of the teacher model it can learn from. In the left plot, the student model’s size is fixed (2 CNN layers). Notice that as the size of the teacher model increases, the accuracy of the student model first increases and then decreases.
For a given size of the teacher model, one can transfer knowledge to student models only up to a certain size, not lower. In the right plot, the teacher model’s size is fixed (10 CNN layers). As the student model’s size decreases, the student model’s accuracy gain over another student model of the same size trained without knowledge distillation first increases and then decreases.
Both plots suggest that the knowledge distillation can only be effective within a specific range of model sizes.
Solution
This can be solved by adding an intermediate model called the teacher assistant:
Step 1: The assistant model learns from the teacher model.
Step 2: The student model learns from the assistant model.
Of course, this introduces an additional training step.
But since development environments are usually flexible, this technique can significantly enhance the performance and efficiency of the final student model.
Moreover, the cost of running the model in production can grow exponentially with demand, but relative to that, training costs still remain low.
Results
The efficacy is evident from the image below:
NOKD → Training the student model directly.
BLKD → Training the student model from the teacher model.
TAKD → Training the student model using the teacher assistant.
In all cases, utilizing the teacher assistant works better than the other two approaches.
Here are the model configurations in the above case:
In this approach, the assistant model can be significantly smaller in size than the teacher model. As shown in the above image, the assistant model is more than 50% smaller than the teacher model.
On a high level, here’s what this approach would look like when implemented:
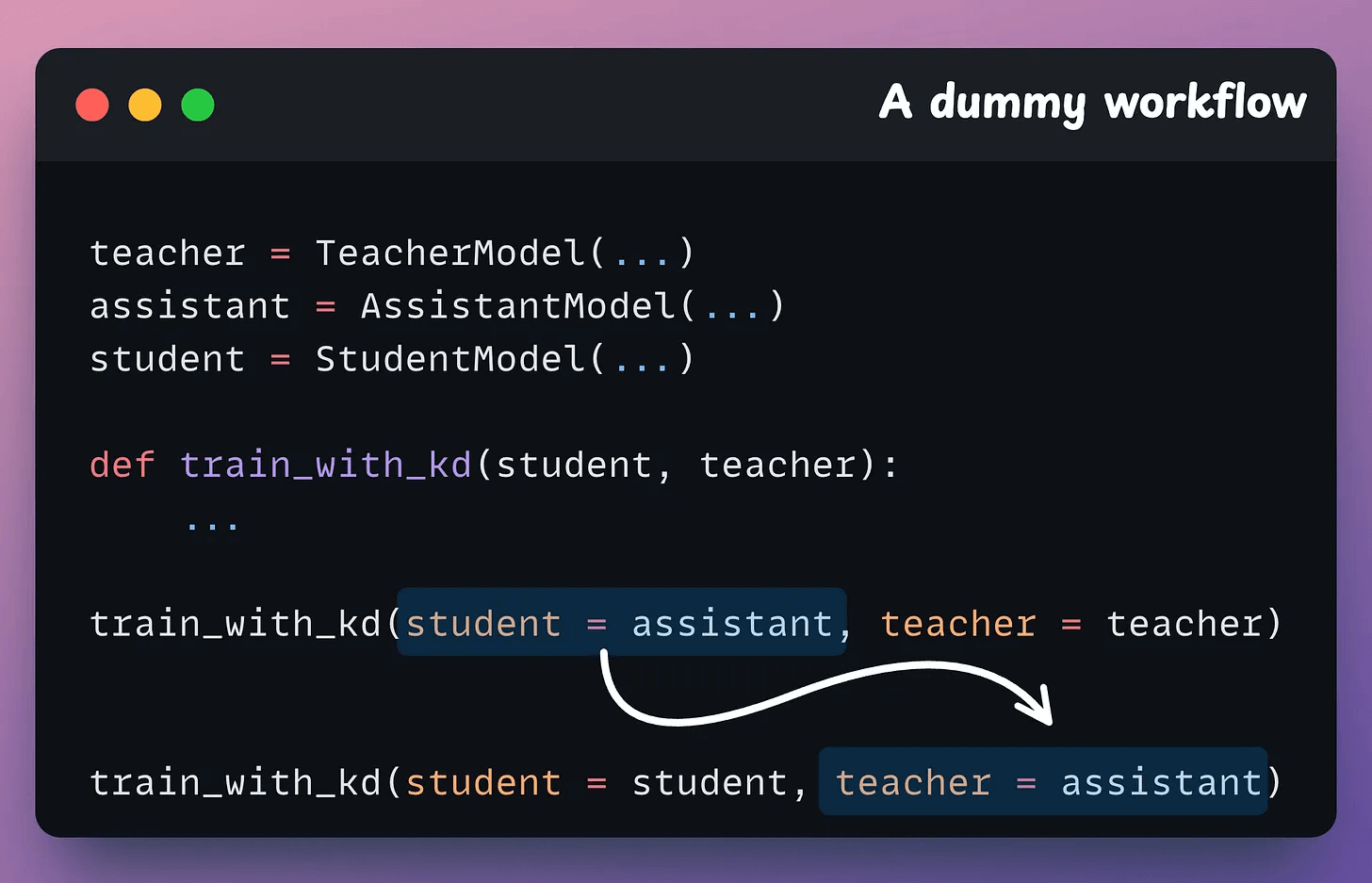
train_with_kd
is assumed to be a user-defined function that trains a student model from a teacher model.That said, if ideas related to production and deployment intimidate you, here’s a quick roadmap to upskill you (assuming you know how to train a model):
First, you would have to compress the model and productionize it. Read these guides:
Model Compression: A Critical Step Towards Efficient Machine Learning.
PyTorch Models Are Not Deployment-Friendly! Supercharge Them With TorchScript.
If you use sklearn, here’s a guide that teaches you to optimize models like decision trees with tensor operations: Sklearn Models are Not Deployment Friendly! Supercharge Them With Tensor Computations.
Next, you move to deployment. Here’s a beginner-friendly hands-on guide that teaches you how to deploy a model, manage dependencies, set up the model registry, etc.: Deploy, Version Control, and Manage ML Models Right From Your Jupyter Notebook with Modelbit.
Although you would have tested the model locally, it is still wise to test it in production. There are risk-free (or low-risk) methods to do that. Read this to learn them: 5 Must-Know Ways to Test ML Models in Production (Implementation Included).
Here’s the paper we discussed today: Improved Knowledge Distillation via Teacher Assistant.
👉 Over to you: What are some other ways to compress ML models?
Thanks for reading!