Knowledge Distillation with Teacher Assistant for Model Compression
A better and intuitive technique to model compression.
Knowledge distillation is quite commonly used to compress large ML models after training.
Today, I want to discuss a “Teacher Assistant” method used to improve this technique. I recently read about this in a research paper.
Let’s begin!
What is knowledge distillation?
As discussed earlier in this newsletter, the idea is to train a smaller/simpler model (called the “student” model) that mimics the behavior of a larger/complex model (called the “teacher” model):
This involves two steps:
Train the teacher model as we typically would.
Train a student model that matches the output of the teacher model.
If we compare it to an academic teacher-student scenario, the student may not be as performant as the teacher. But with consistent training, a smaller model may get (almost) as good as the larger one.
A classic example of a model developed in this way is DistillBERT. It is a student model of BERT.
DistilBERT is approximately 40% smaller than BERT, which is a massive difference in size.
Still, it retains approximately 97% of the BERT’s capabilities.
If you want to get into more detail about the implementation, we covered it here, with 5 more techniques for model compression: Model Compression: A Critical Step Towards Efficient Machine Learning.
An issue with knowledge distillation
Quite apparently, we always desire the student model to be as small as possible while retaining the maximum amount of knowledge, correct?
However, in practice, two things are observed:
For a given size of the student model, there is a limit on the size of the teacher model it can learn from.
For a given size of the teacher model, one can effectively transfer knowledge to student models only up to a certain size, not lower.
This is evident from the plots below on the CIFAR-10 dataset:
In the left plot, the student model’s size is fixed (2 CNN layers). Here, we notice that as the size of the teacher model increases, the accuracy of the student model first increases and then decreases.
In the right plot, the teacher model’s size is fixed (10 CNN layers). As the student model’s size decreases, the student model’s accuracy gain over another student model of the same size trained without knowledge distillation first increases and then decreases.
Both plots suggest that the knowledge distillation can only be effective within a specific range of model sizes.
Solution
A simple technique to address this is by introducing an intermediate model called the “teacher assistant.”
The process is simple, as depicted in the image below:
Step 1: The assistant model learns from the teacher model using the standard knowledge distillation process.
Step 2: The student model learns from the assistant model using the standard knowledge distillation process.
Yet again, this resonates with an actual academic setting—a teaching assistant often acts as an intermediary between a highly skilled professor and an amateur junior student.
The teaching assistant has a closer understanding of the professor’s complex material and is simultaneously more familiar with the learning pace of the junior students and their beginner mindset.
Of course, this adds a layer of additional training in the model building process.
However, given that development environments are usually not restricted in terms of the available computing resources, this technique can significantly enhance the performance and efficiency of the final student model.
Moreover, the cost of running the model in production can grow exponentially with demand, but relative to that, training costs still remain low, so the idea is indeed worth pursuing.
Results
The efficacy is evident from the image below:
NOKD → Training a model of student’s size directly.
BLKD → Training the student model from the teacher model.
TAKD → Training the student model using the teacher assistant.
In all cases, utilizing the teacher assistant works better than the other two approaches.
Here are the model configurations in the above case:
One interesting observation in these results is that the assistant model does not have to be significantly similar in size to the teacher model. For instance, in the above image, the assistant model is more than 50% smaller in all cases than the teacher model.
The authors even tested this.
Consider the table below, wherein the size of the assistant model is varied while the size of the student and teacher models is fixed. The numbers reported are the accuracy of the student model in each case.
As depicted above, the difference in accuracy with a 56-layer assistant model is quite close (and even better once) to the accuracy with a 4 times smaller assistant model (14-layers).
Impressive, right?
Since we have already covered the implementation before (read this for more info), here’s a quick summary of what this would look like:
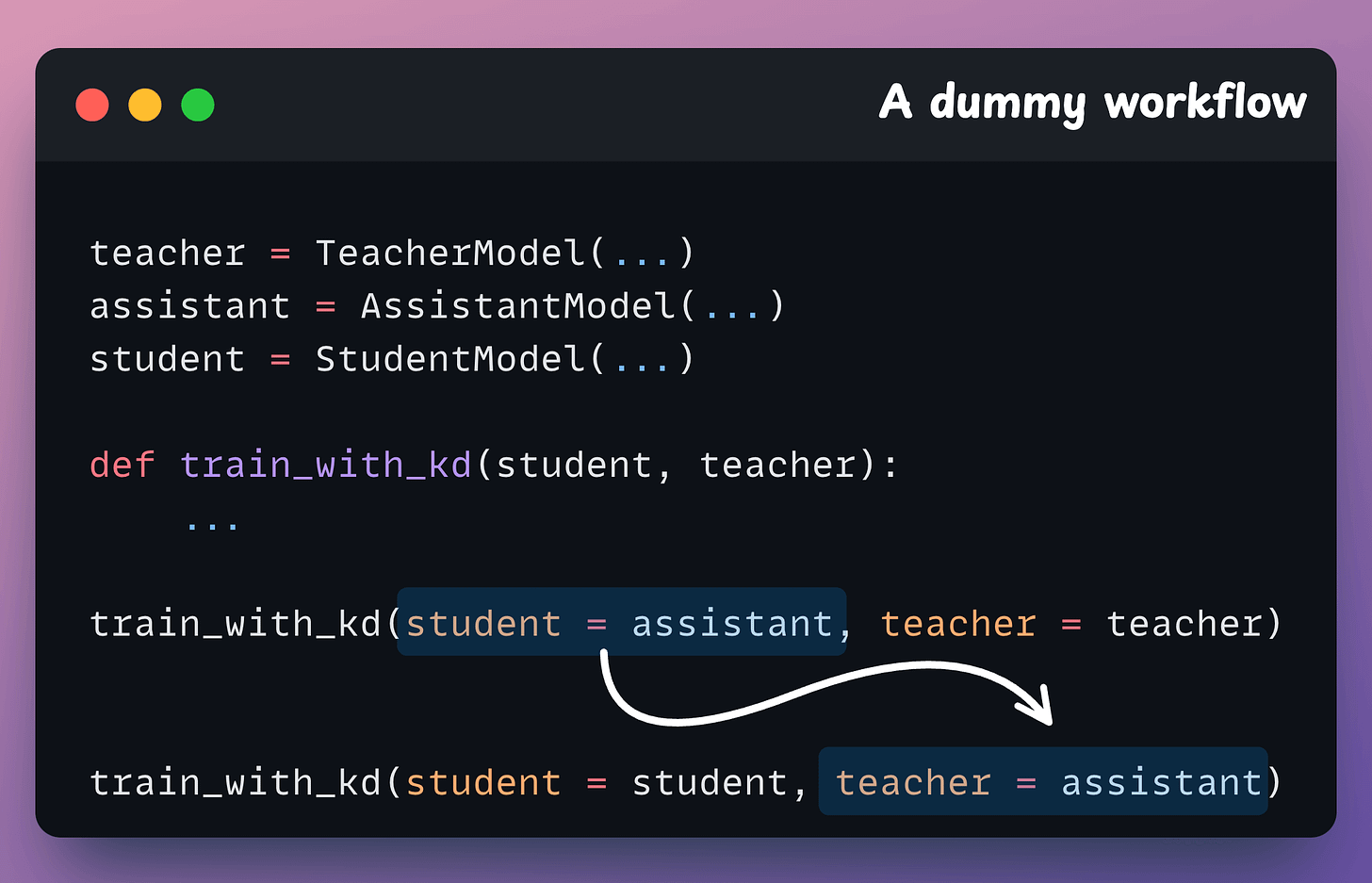
train_with_kd
is assumed to be a user-defined function that trains a student model from a teacher model.Hope you learned something new today!
That said, if ideas related to production and deployment intimidate you, here’s a quick roadmap to upskill you (assuming you know how to train a model):
First, you would have to compress the model and productionize it. Read these guides:
Model Compression: A Critical Step Towards Efficient Machine Learning.
PyTorch Models Are Not Deployment-Friendly! Supercharge Them With TorchScript.
If you use sklearn, here’s a guide that teaches you to optimize models like decision trees with tensor operations: Sklearn Models are Not Deployment Friendly! Supercharge Them With Tensor Computations.
Next, you move to deployment. Here’s a beginner-friendly hands-on guide that teaches you how to deploy a model, manage dependencies, set up model registry, etc.: Deploy, Version Control, and Manage ML Models Right From Your Jupyter Notebook with Modelbit.
Although you would have tested the model locally, it is still wise to test it in production. There are risk-free (or low-risk) methods to do that. Read this to learn them: 5 Must-Know Ways to Test ML Models in Production (Implementation Included).
Here’s the paper we discussed today: Improved Knowledge Distillation via Teacher Assistant.
👉 Over to you: What are some other ways to compress ML models.
Are you overwhelmed with the amount of information in ML/DS?
Every week, I publish no-fluff deep dives on topics that truly matter to your skills for ML/DS roles.
For instance:
Conformal Predictions: Build Confidence in Your ML Model’s Predictions
Quantization: Optimize ML Models to Run Them on Tiny Hardware
5 Must-Know Ways to Test ML Models in Production (Implementation Included)
8 Fatal (Yet Non-obvious) Pitfalls and Cautionary Measures in Data Science
Implementing Parallelized CUDA Programs From Scratch Using CUDA Programming
You Are Probably Building Inconsistent Classification Models Without Even Realizing
And many many more.
Join below to unlock all full articles:
SPONSOR US
Get your product in front of 87,000 data scientists and other tech professionals.
Our newsletter puts your products and services directly in front of an audience that matters — thousands of leaders, senior data scientists, machine learning engineers, data analysts, etc., who have influence over significant tech decisions and big purchases.
To ensure your product reaches this influential audience, reserve your space here or reply to this email to ensure your product reaches this influential audience.