Our team is growing!!
This week, Akshay Pachaar joined me as a co-founder in the Daily Dose of Data Science.
Akshay has made some incredible contributions to the AI community, especially over X, and after his time at Lightning.AI, he decided to conduct independent AI research and write about it.
I couldn’t have found anyone better to serve you through this newsletter.
Going ahead, we both will be working together to bring you these daily newsletter issues and the deep dives, along with what you read on social.
If you have some specific topics around AI/ML engineering, data science, etc., that you want us to cover, feel free to reply to this email.
Let’s get to today’s issue now!
Mixed precision training
Yesterday, we learned about activation checkpointing to optimize neural network training.
If you are new here or missed reading about it, here’s a summary of the technique:
Today, let’s learn about mixed precision training, which is another pretty popular technique to optimize neural network training.
Let’s begin!
While mixed precision training is one way, we covered 15 techniques to optimize neural network training here: 15 Ways to Optimize Neural Network Training (With Implementation).
Motivation
Typical deep learning libraries are really conservative when it comes to assigning data types.
The data type assigned by default is usually 64-bit or 32-bit, when there is also scope for 16-bit, for instance. This is also evident from the code below:
As a result, we are not entirely optimal at efficiently allocating memory.
Of course, this is done to ensure better precision in representing information.
However, this precision always comes at the cost of additional memory utilization, which may not be desired in all situations.
In fact, it is also observed that many tensor operations, especially matrix multiplication, are much faster when we operate under smaller precision data types than larger ones, as demonstrated below:
Moreover, since float16
is only half the size of float32
, its usage reduces the memory required to train the network.
This also allows us to train larger models, train on larger mini-batches (resulting in even more speedup), etc.
Mixed precision training is a pretty reliable and widely adopted technique in the industry to achieve this.
As the name suggests, the idea is to employ lower precision float16
(wherever feasible, like in convolutions and matrix multiplications) along with float32
— that is why the name “mixed precision.”
This is a list of some models I found that were trained using mixed precision:
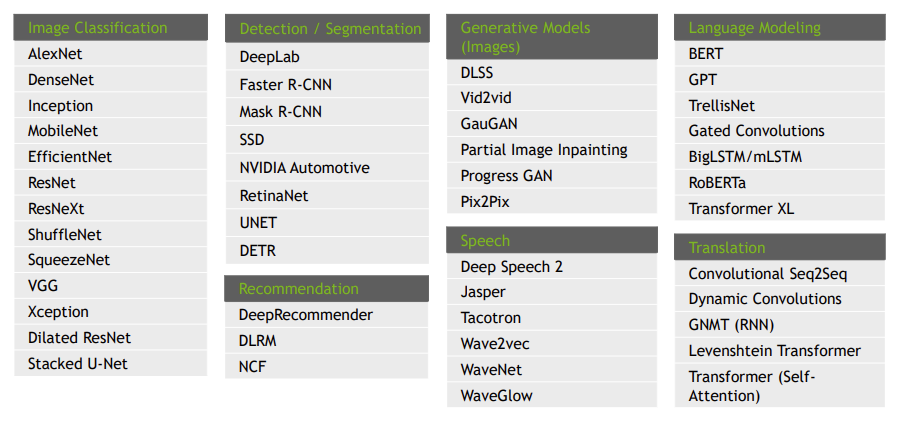
It’s pretty clear that mixed precision training is much more popularly used, but we don’t get to hear about it often.
Before we get into the technical details…
From the above discussion, it must be clear that as we use a low-precision data type (float16
), we might unknowingly introduce some numerical inconsistencies and inaccuracies.
To avoid them, there are some best practices for mixed precision training that I want to talk about next, along with the code.
Mixed precision training in PyTorch and Best Practices
Leveraging mixed precision training in PyTorch requires a few modifications in the existing network training implementation.
Consider this is our current PyTorch model training implementation:
The first thing we introduce here is a scaler
object that will scale the loss value:
We do this because, at times, the original loss value can be so low, that we might not be able to compute gradients in float16
with full precision.
Such situations may not produce any update to the model’s weights.
Scaling the loss to a higher numerical range ensures that even small gradients can contribute to the weight updates.
But these minute gradients can only be accommodated into the weight matrix when the weight matrix itself is represented in high precision, i.e., float32
.
Thus, as a conservative measure, we tend to keep the weights in float32
.
That said, the loss scaling step is not entirely necessary because, in my experience, these little updates typically appear towards the end stages of the model training.
Thus, it can be fair to assume that small updates may not drastically impact the model performance.
But don’t take this as a definite conclusion, so it’s something that I want you to validate when you use mixed precision training.
Moving on, as the weights (which are matrices) are represented in float32
, we can not expect the speedup from representing them in float16
, if they remain this way:
To leverage these flaot16
-based speedups, here are the steps we follow:
We make a
float16
copy of weights during the forward pass.Next, we compute the loss value in
float32
and scale it to have more precision in gradients, which works infloat16
.The reason we compute gradients in float16 is because, like forward pass, gradient computations also involve matrix multiplications.
Thus, keeping them in
float16
can provide additional speedup.
Once we have computed the gradients in
float16
, the heavy matrix multiplication operations have been completed. Now, all we need to do is update the original weight matrix, which is infloat32
.Thus, we make a
float32
copy of the above gradients, remove the scale we applied in Step 2, and update thefloat32
weights.Done!
The mixed-precision settings in the forward pass are carried out by the torch.autocast()
context manager:
Now, it’s time to handle the backward pass.
Line 13 →
scaler.scale(loss).backward()
: Thescaler
object scales the loss value andbackward()
is called to compute the gradients.Line 14 →
scaler.step(opt)
: Unscale gradients and update weights.Line 15 →
scaler.update()
: Update the scale for the next iteration.Line 16 →
opt.zero_grad()
: Zero gradients.
Done!
The efficacy of mixed precision scaling over traditional training is evident from the image below:
Mixed precision training is over 2.5x faster than conventional training.
Isn’t that cool?
If the above code is a bit intimidating and tedious, PyTorch Lightning makes it extremely simple, along with several other cool features. We covered this here: A Detailed and Beginner-Friendly Introduction to PyTorch Lightning: The Supercharged PyTorch.
Also, we covered 15 techniques to optimize neural network training here: 15 Ways to Optimize Neural Network Training (With Implementation).
👉 Over to you: What are some other reliable ways to speed up machine learning model training?
P.S. For those wanting to develop “Industry ML” expertise:
At the end of the day, all businesses care about impact. That’s it!
Can you reduce costs?
Drive revenue?
Can you scale ML models?
Predict trends before they happen?
We have discussed several other topics (with implementations) in the past that align with such topics.
Here are some of them:
Learn techniques to run large models on small devices: Quantization: Optimize ML Models to Run Them on Tiny Hardware
Learn how to generate prediction intervals or sets with strong statistical guarantees for increasing trust: Conformal Predictions: Build Confidence in Your ML Model’s Predictions.
Learn how to identify causal relationships and answer business questions: A Crash Course on Causality – Part 1
Learn how to scale ML model training: A Practical Guide to Scaling ML Model Training.
Learn techniques to reliably roll out new models in production: 5 Must-Know Ways to Test ML Models in Production (Implementation Included)
Learn how to build privacy-first ML systems: Federated Learning: A Critical Step Towards Privacy-Preserving Machine Learning.
Learn how to compress ML models and reduce costs: Model Compression: A Critical Step Towards Efficient Machine Learning.
All these resources will help you cultivate key skills that businesses and companies care about the most.
SPONSOR US
Get your product in front of 104k+ data scientists and other tech professionals.
Our newsletter puts your products and services directly in front of an audience that matters — thousands of leaders, senior data scientists, machine learning engineers, data analysts, etc., who have influence over significant tech decisions and big purchases.
To ensure your product reaches this influential audience, reserve your space here or reply to this email to ensure your product reaches this influential audience.
Excellent content
Very well explained!